Our Services
AI Solutions and Products
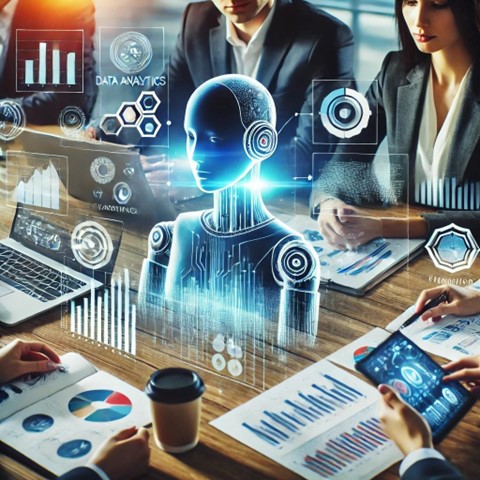
- Predictive Analytics
- Computer Vision
- Natural Language Processing
- Intelligent Automation
These AI solutions and products enable organizations to harness the power of AI to enhance decision-making, automate complex processes, and create innovative applications that drive business growth and efficiency.
Predictive Analytics
Predictive Analytics is an AI-driven solution that leverages statistical algorithms, machine learning, and data mining techniques to analyze historical data and make predictions about future events. This solution is widely used across industries to forecast trends, anticipate customer behavior, and optimize business processes.
Here’s how predictive analytics works:
Data Collection and Preparation
Predictive analytics begins with gathering and processing large datasets from various sources, such as customer transactions, social media activity, sensor data, and more. This data is cleaned, normalized, and structured to ensure accuracy and consistency.
Model Building
AI algorithms, including machine learning models like decision trees, neural networks, and regression models, are used to analyze the historical data. These models learn patterns and relationships within the data that can be used to predict future outcomes.
Feature Engineering
This step involves selecting and transforming relevant variables (features) from the data that the AI model will use to make predictions. Feature engineering is crucial for improving the model’s accuracy and performance.
Prediction Generation
Once the model is trained, it is applied to new data to generate predictions. For example, predictive analytics can forecast sales trends, predict customer churn, estimate demand for products, or assess credit risk.
Continuous Learning
I models in predictive analytics are continuously updated with new data, allowing them to adapt to changing conditions and improve over time. This ensures that predictions remain accurate and relevant.
Business Applications
Predictive analytics is used in various business scenarios, such as optimizing inventory management, personalizing marketing campaigns, improving customer retention, and reducing operational risks. It helps organizations make data-driven decisions and gain a competitive advantage.
Computer Vision
Computer Vision is an AI-driven technology that enables machines to interpret and understand visual information from the world, such as images and videos. This technology mimics human vision and is used in various applications, ranging from facial recognition to autonomous vehicles.
Here’s how computer vision works:
Image Acquisition
The process begins with capturing images or video frames through cameras or sensors. These visual inputs serve as the raw data that the AI system will analyze.
Image Processing
The captured images are processed using various techniques such as filtering, edge detection, and color correction to enhance their quality and extract relevant features. This step prepares the images for further analysis.
Object Detection and Recognition
AI algorithms, such as convolutional neural networks (CNNs), are used to detect and recognize objects within the images. This involves identifying patterns and features that correspond to specific objects, such as faces, vehicles, or text.
Segmentation and Classification
In this step, the image is divided into segments, and each segment is classified into different categories. For example, in medical imaging, computer vision can segment an MRI scan into different tissue types and classify them as healthy or diseased.
Motion and Tracking Analysis
In video analysis, computer vision can track moving objects over time. This is used in applications like surveillance, where the system monitors and tracks people or vehicles in real-time.
Pattern Recognition
Computer vision systems can recognize complex patterns and anomalies in images. For example, in manufacturing, it can detect defects in products on an assembly line.
Applications
Computer vision is widely used in industries such as healthcare (for diagnostic imaging), automotive (for autonomous driving), retail (for visual search and inventory management), and security (for facial recognition and surveillance).
Natural Language Processing (NLP)
Natural Language Processing (NLP) is an AI-driven solution that enables machines to understand, interpret, and generate human language. NLP combines computational linguistics, machine learning, and deep learning to process natural language in both written and spoken forms.
Here’s how NLP works:
Text Preprocessing
NLP starts with preprocessing the text data, which includes tasks like tokenization (splitting text into words or phrases), stemming (reducing words to their root form), and removing stop words (common words like “the” or “is” that do not contribute much meaning).
Syntactic and Semantic Analysis
NLP systems analyze the syntax (structure) and semantics (meaning) of the text. Syntactic analysis involves parsing sentences to understand grammatical relationships, while semantic analysis focuses on extracting the meaning of words and phrases.
Sentiment Analysis
LP algorithms can detect and analyze the sentiment expressed in the text, categorizing it as positive, negative, or neutral. This is commonly used in social media monitoring, customer feedback analysis, and brand reputation management.
Named Entity Recognition (NER)
NLP identifies and classifies entities in the text, such as names of people, organizations, locations, dates, and more. NER is used in information extraction, content categorization, and knowledge graph construction.
Machine Translation
NLP powers machine translation systems that automatically translate text from one language to another. Examples include Google Translate and real-time translation services in communication apps.
Speech Recognition and Generation
NLP enables voice assistants like Siri, Alexa, and Google Assistant to understand spoken language and respond with appropriate actions or spoken responses. Speech-to-text and text-to-speech technologies are integral to these applications.
Text Summarization and Generation
NLP can automatically summarize long documents or generate new text based on input data. This is used in applications like content creation, report generation, and chatbots.
Applications
NLP is used in various domains, including customer service (chatbots), healthcare (medical record analysis), legal (contract analysis), and finance (automated trading and sentiment analysis).
Intelligent Automation
Intelligent Automation combines AI and automation technologies to create systems that can perform complex tasks with minimal human intervention. It goes beyond traditional automation by incorporating AI capabilities like machine learning, NLP, and computer vision to make decisions, adapt to new situations, and improve over time.
Here’s how intelligent automation works:
Process Automation
Intelligent automation starts with automating repetitive and rule-based tasks using robotic process automation (RPA). RPA bots can interact with digital systems, handle data entry, process transactions, and perform other routine tasks.
AI Integration
AI technologies are integrated into the automation process to enable more complex decision-making and cognitive tasks. For example, machine learning algorithms can be used to analyze data and predict outcomes, while NLP can process unstructured text data.
End-to-End Automation
Intelligent automation can automate entire workflows, from start to finish. For example, in customer service, an automated system can handle a customer query, retrieve relevant information, and provide a personalized response, all without human intervention.
Adaptive Systems
Unlike traditional automation, intelligent automation systems can learn from their experiences and adapt to changing conditions. Machine learning models are continuously trained on new data, allowing the system to improve its accuracy and performance over time.
Human-AI Collaboration
Intelligent automation allows for seamless collaboration between humans and machines. AI systems can handle routine tasks, freeing up human workers to focus on higher-value activities that require creativity, problem-solving, and critical thinking.
Predictive Maintenance
In industries like manufacturing, intelligent automation is used for predictive maintenance. AI models analyze sensor data from machines to predict when maintenance is needed, reducing downtime and preventing equipment failures.
Compliance and Risk Management
Intelligent automation ensures compliance with regulations by automatically monitoring and reporting on processes. AI algorithms can also identify potential risks and suggest corrective actions, improving overall governance.
Applications
Intelligent automation is used in various sectors, including finance (automated trading and fraud detection), healthcare (automated diagnostics and patient management), supply chain (inventory management and logistics optimization), and customer service (chatbots and virtual assistants).